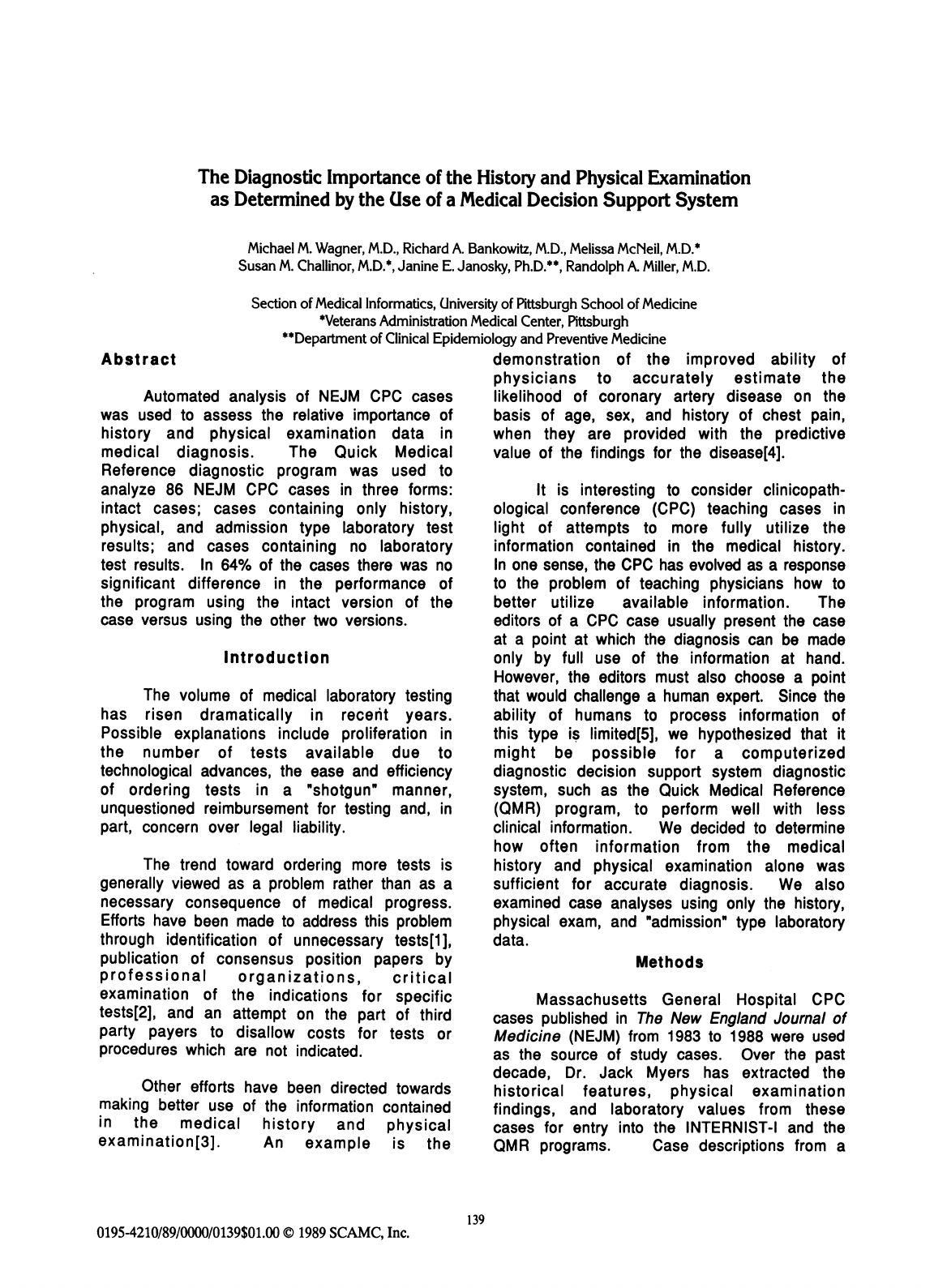
The
Diagnostic
Importance
of
the
History
and
Physical
Examination
as
Determined
by
the
Use
of
a
Medical
Decision
Support
System
Michael
M.
Wagner,
M.D.,
Richard
A.
Bankowitz,
M.D.,
Melissa
McNeil,
M.D.*
Susan
M.
Challinor,
M.D.*,
Janine
E.
Janosky,
Ph.D.**,
Randolph
A.
Miller,
M.D.
Section
of
Medical
Informatics,
University
of
Pittsburgh
School
of
Medicine
*Veterans
Administration
Medical
Center,
Pittsburgh
**Departrnent
of
Clinical
Epidemiology
and
Preventive
Medicine
Abstract
Automated
analysis
of
NEJM
CPC
cases
was
used
to
assess
the
relative
importance
of
history
and
physical
examination
data
in
medical
diagnosis.
The
Quick
Medical
Reference
diagnostic
program
was
used
to
analyze
86
NEJM
CPC
cases
in
three
forms:
intact
cases;
cases
containing
only
history,
physical,
and
admission
type
laboratory
test
results;
and
cases
containing
no
laboratory
test
results.
In
64%
of
the
cases
there
was
no
significant
difference
in
the
performance
of
the
program
using
the
intact
version
of
the
case
versus
using
the
other
two
versions.
Introduction
The
volume
of
medical
laboratory
testing
has
risen
dramatically
in
recent
years.
Possible
explanations
include
proliferation
in
the
number
of
tests
available
due
to
technological
advances,
the
ease
and
efficiency
of
ordering
tests
in
a
"shotgun'
manner,
unquestioned
reimbursement
for
testing
and,
in
part,
concern
over
legal
liability.
The
trend
toward
ordering
more
tests
is
generally
viewed
as
a
problem
rather
than
as
a
necessary
consequence
of
medical
progress.
Efforts
have
been
made
to
address
this
problem
through
identification
of
unnecessary
tests[1],
publication
of
consensus
position
papers
by
professional
organizations,
critical
examination
of
the
indications
for
specific
tests[2],
and
an
attempt
on
the
part
of
third
party
payers
to
disallow
costs
for
tests
or
procedures
which
are
not
indicated.
Other
efforts
have
been
directed
towards
making
better
use
of
the
information
contained
in
the
medical
history
and
physical
examination[3].
An
example
is
the
demonstration
of
the
improved
ability
of
physicians
to
accurately
estimate
the
likelihood
of
coronary
artery
disease
on
the
basis
of
age,
sex,
and
history
of
chest
pain,
when
they
are
provided
with
the
predictive
value
of
the
findings
for
the
disease[4].
It
is
interesting
to
consider
clinicopath-
ological
conference
(CPC)
teaching
cases
in
light
of
attempts
to
more
fully
utilize
the
information
contained
in
the
medical
history.
In
one
sense,
the
CPC
has
evolved
as
a
response
to
the
problem
of
teaching
physicians
how
to
better
utilize
available
information.
The
editors
of
a
CPC
case
usually
present
the
case
at
a
point
at
which
the
diagnosis
can
be
made
only
by
full
use
of
the
information
at
hand.
However,
the
editors
must
also
choose
a
point
that
would
challenge
a
human
expert.
Since
the
ability
of
humans
to
process
information
of
this
type
is
limited[5],
we
hypothesized
that
it
might
be
possible
for
a
computerized
diagnostic
decision
support
system
diagnostic
system,
such
as
the
Quick
Medical
Reference
(QMR)
program,
to
perform
well
with
less
clinical
information.
We
decided
to
determine
how
often
information
from
the
medical
history
and
physical
examination
alone
was
sufficient
for
accurate
diagnosis.
We
also
examined
case
analyses
using
only
the
history,
physical
exam,
and
"admission"
type
laboratory
data.
Methods
Massachusetts
General
Hospital
CPC
cases
published
in
The
New
England
Journal
of
Medicine
(NEJM)
from
1983
to
1988
were
used
as
the
source
of
study
cases.
Over
the
past
decade,
Dr.
Jack
Myers
has
extracted
the
historical
features,
physical
examination
findings,
and
laboratory
values
from
these
cases
for
entry
into
the
INTERNIST-I
and
the
QMR
programs.
Case
descriptions
from
a
139
0195-4210/89/0000/0139$01.00
©
1989
SCAMC,
Inc.